by Peter Coldicott
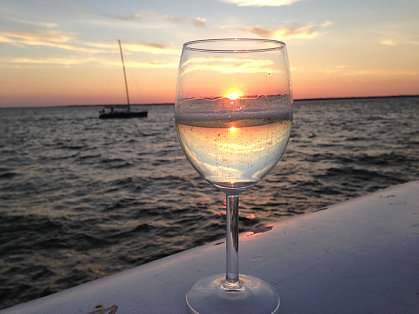
Trustworthy (less-biased) AI starts with strong Data Governance.
Artificial Intelligence (AI) systems are in the news a lot right now, and apart from the many articles put forward by the builders of these systems on how great they are going to make things, many of the articles are about the lack of trust in such systems. In those less-positive articles, there is discussion of bias, transparency, and job losses as some of the major reasons not to trust results derived by AI.
I believe there are several factors worthy of discussion here, but the one that seems to invoke the most distrust in the technology is bias. Bias is an interesting phenomenon. Bias has always been present, whether conscious or unconscious, as humans, we are to some degree all plagued with it. However, bias in AI terms is normally a question of the contents of the data used to train the AI. I will point out here that it is my personal belief that as things stand AI’s should never MAKE decisions, they should only inform and provide input to human decision makers. If you can accept that, then my first question would be, are the results returned by the AI based system more, or less biased than if a human was providing that data? Second, how much do you trust the data being used to train the AI.
I am not saying that we should not worry about bias in AI systems, what I am saying is that we need to understand where the potential bias is and how it affects the resultant AI. In some instances, the bias in the data is obvious, for example only men were included in the data when the AI will also process data about women. However, often the bias is much more subtle and difficult to identify.
That is why it is important to have a deep understanding of the training data, how complete it is, where it comes from, how reliable it is, and so on. These are all questions that should be easy to get answers to, unfortunately in the main it isn’t. Disciplined data management can help immensely with these kind of questions by providing metadata about the data. Gathering that (meta) data does, of course, present its own data management issues and so we need a system to store, manage, update metadata and a way to search for what is needed, one way to do that is with the Egeria open-source technology.
In my opinion you need three things in place before leaping into the AI world.
- Have a robust and functioning metadata management system operational and collecting metadata from at least the systems relevant to the area you are considering applying AI to.
- A well tested and understood set of analytics appropriate to the business area and use case(s) you are going to apply AI to.
- A rigorous and continuous testing regime that tests the boundaries of your algorithms and the reasonableness of the results. Often one of the things that is forgotten is that an AI can produce reasonable answers when the range of the input conditions is within the range of the training data…if the real-world parameters are outside of the boundaries of the training data then you can’t trust the results.
With these three pre-requisites in place, you can DEFEND your use of AI, EXPLAIN the results you are getting and EXPRESS CONFIDENCE in the results, which will in turn help instill confidence in others.
We are happy to help you on your data management journey: pdr-associates.com